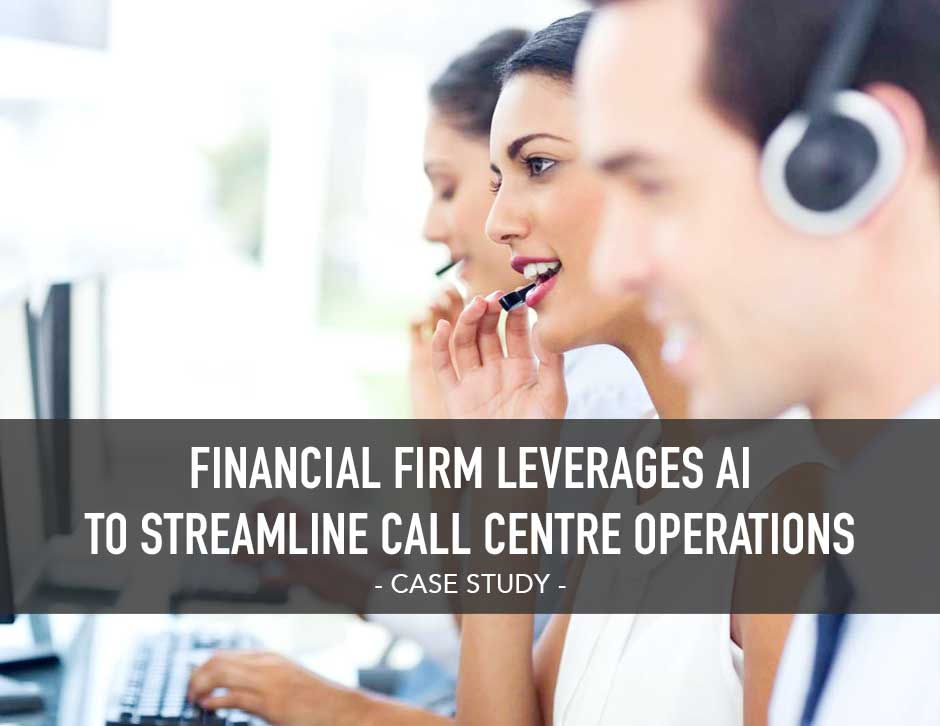
A Spanish financial firm that focuses on asset management, investment services and insurance operates a call centre which allows clients to get quick information and updates about their investments and other financial services. The company has a long history and a reputation of providing top-tier services to its clients, where the call centre plays an important role.
The call centre has a double purpose for current clients; primarily to provide information, but also to offer new financial products, i.e. cross-selling. Non-clients can also call and are channelled to the sales team for onboarding.
Although the average incoming calls per day are over 800, the call centre frequently experiences more than 1000 calls per day and even up to 1500 occasionally. The company has received a number of complaints from clients who are unable to reach agents by phone, as well as long waiting times with some even staying on the line for 60 minutes.
The Challenge
The company identified three operational challenges within the call centre and approached Sumo Analytics for advising on advanced analytics solutions:
The company utilises sophisticated call centre software which has a built-in forecasting tool that forecasts the number of inbound calls, which helps with understanding staffing requirements. However, the forecasts lack accuracy which is often the case with out-of-the-box software. This led to poor planning where the call centre was often under- or over staffed. When understaffed, it was not uncommon that service level was only between 50-60%. As the management of the call centre tried to respond to client complaints about poor service, adding more staff turned out to be tremendously costly as much of the time operators were inactive during days with low-level of incoming calls.
Survey among clients suggested that many were interested in exploring more financial products from the company. Nevertheless, cross-sales within the call centre were low and didn’t seem to provide much additional value. The company had profiled clients in different categories which were labelled with different service offerings tailored to each category. The call centre operators worked according to this profiling, aiming to offer tailored products and services, but without much success.
The call centre new client onboarding level had been decreasing over the previous years, which had got the senior management believing their financial products were “not interesting enough” or that wrong products were offered to prospects.
The Solution
After initial discussions with the company’s management, we recommended that the company would adopt advanced AI-driven forecasts in order to have an accurate number of incoming calls. We developed sophisticated forecast models that forecast calls for every hour of the day, as well as daily 30-day forecasts, and weekly 52-week forecasts which increased the company’s forecasting accuracy by a staggering 76%.
We utilised RShiny and built a dynamic web application that displays the forecasts and staffing predictions through a modified Erlang Calculator, along with different KPIs for monitoring performance.
The cross-selling challenge was reassessed and eventually we recommended abandoning the customer profiling the company had relied up on. Instead, we built a predictive cross-selling model which helps call centre operators to offer the products most likely to be accepted by each and every customer. The model takes into account current products, call history and interactions with the company, demographics and other data. Once clients call, operators have a list of products the calling client is most likely to be interested in.
A modified version of the same cross-selling model was used when designing predictive sales tool for new clients, which enables the sales team to offer prospective clients financial products more likely to be accepted by them.
The Benefits
With forecast accuracy increasing 76% and a modified Erlang Calculator, staffing shifts has become more accurate than ever with service level hitting 98% without experiencing much overstaffing. Likewise, cross-selling increased significantly as well the level of new client onboarding as the prediction models were able to assist operators and sales teams with more accurate product offering.