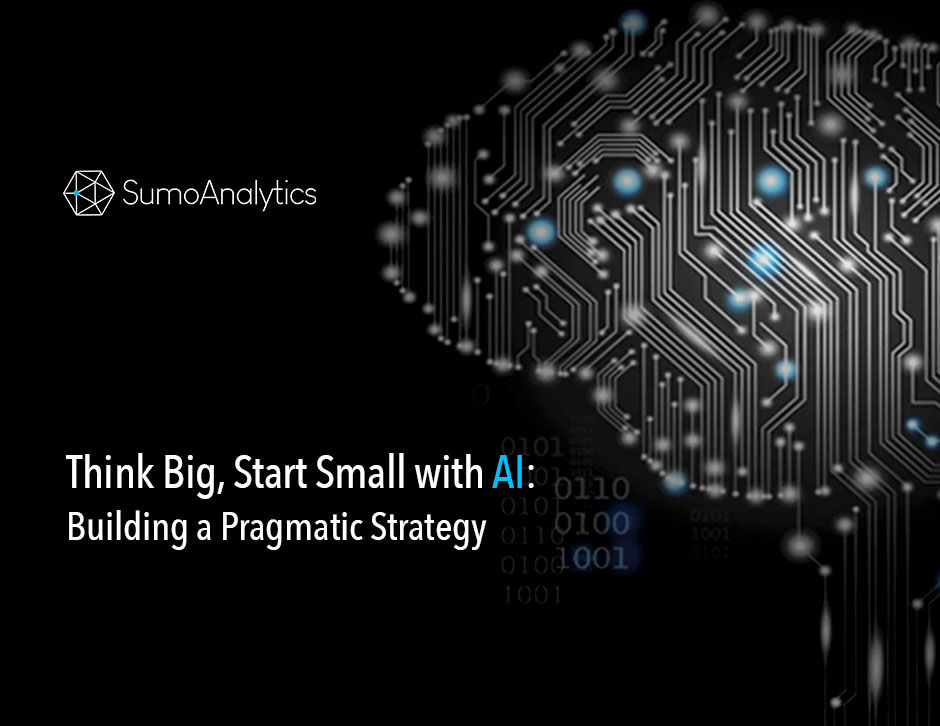
In today's digital age, Artificial Intelligence (AI) is not merely a buzzword; it's a transformative force redefining the boundaries of what businesses can achieve. Across industries, from healthcare to finance and from retail to manufacturing, AI-powered solutions are revolutionizing operations, enhancing customer experiences, and unlocking new avenues of growth.
The current AI landscape is both vast and dynamic. Cutting-edge breakthroughs are happening at an unprecedented pace, with models growing in sophistication and capability. Whether it's through deep learning algorithms that can detect patterns humans can't, natural language processing that can engage customers seamlessly, generative AI and everything surrounding large language models (LLMs), or predictive analytics that can forecast market trends with astonishing accuracy, AI's potential seems boundless.
Yet, with such immense possibilities at our fingertips, a strategic paradox emerges for businesses eager to harness the power of AI: the challenge of balancing aspirational goals with pragmatic beginnings. The enthusiasm to incorporate AI in every facet of the business, driven by its groundbreaking successes, can be overwhelming. But here's where the age-old adage, "Rome wasn't built in a day," rings particularly true.
Thinking big with AI is not just about envisioning its vast potential but understanding the intricate web of implementations required to achieve that vision. Conversely, starting small does not mean limiting aspirations; rather, it's a recognition that the path to AI mastery is a journey. It's an iterative process of learning, adapting, and scaling. A measured approach—where companies initiate with focused, manageable projects—ensures that the foundation is solid, risks are mitigated, and the entire organization moves forward in harmony with the AI transformation.
Thus, as we delve deeper into the realm of AI strategy, remember this: Dreaming big opens the doors to what's possible, but starting small ensures those doors lead to success.
Setting the Vision: The 'Big Think'
Embarking on the AI journey without a clear vision is like setting sail without a compass: while you might catch favorable winds, there's no guarantee you'll reach your desired destination. Establishing a strong AI vision is pivotal not just for direction but also to inspire, motivate, and guide your entire organization towards a unified goal.
The Significance of Long-term AI Objectives
Understanding your long-term AI objectives is crucial for multiple reasons:
Strategic Alignment: Clearly defined objectives ensure that AI initiatives are in sync with the broader business goals. Whether it's boosting sales, improving customer engagement, or streamlining operations, your AI projects should resonate with what your business ultimately aims to achieve.
Resource Allocation: Knowing what you want to accomplish helps in efficiently allocating resources. Whether it's human capital, technology investments, or time—having clear objectives ensures that you're not spreading yourself too thin or investing too heavily in one area at the expense of another.
Performance Metrics: With a clear vision, you can establish tangible metrics to measure the success of your AI initiatives. These can range from ROI to more qualitative measures such as improved customer satisfaction or employee productivity.
Questions to Guide Vision Setting
When sketching out the AI roadmap for your business, introspection is key. Here are some guiding questions to steer the process:
Where do you see AI in your company in 5 years? Envisioning the medium-term can strike the right balance between what's aspirational and what's achievable. Perhaps you see AI-powered chatbots handling a majority of customer queries or predictive analytics shaping product development. This vision helps bridge the gap between present capabilities and future aspirations.
Which core areas of your business can most benefit from AI? Not all areas of a business will benefit from AI equally. Some might require a more immediate AI integration due to potential returns, while others might benefit from a longer, more iterative approach. Is it the supply chain that needs predictive optimization? Or is the marketing department craving insights from deep data analysis? Identifying these core areas helps in setting both priorities and expectations.
In conclusion, the 'Big Think' is not about lofty, unreachable dreams but about clarity, focus, and direction. It's about understanding where you want to go with AI and charting a course that's both ambitious and anchored in the realities of your business landscape.
The Dangers of Diving in Headfirst
The allure of AI's transformative potential can often lead companies down a path of overzealous adoption. Fueled by the successes of tech giants and the constant buzz surrounding AI breakthroughs, there's a palpable excitement to integrate AI everywhere, all at once. However, without a strategic and phased approach, such endeavors can quickly become cautionary tales.
Challenges of Simultaneous Large-Scale AI Implementations
Complexity Amplification: As the scale of AI implementation grows, so does its complexity. Juggling multiple projects can lead to overlapping objectives, creating a foggy landscape where the direction and purpose of each project become unclear.
Operational Overload: With multiple AI projects running simultaneously, the day-to-day operations can be severely strained. There's a real risk of regular business processes being disrupted, leading to inefficiencies and reduced productivity.
Inconsistent Data Management: Handling data for one AI project can be challenging enough; doing so for multiple projects can be a nightmare. Without uniform standards, there's a danger of creating silos, leading to inconsistent, and sometimes even contradictory, insights.
Potential Pitfalls
Over-extension of Resources: Diving into large-scale AI adoption can strain resources—both in terms of finances and manpower. This can lead to a scenario where there's much in motion but too little in-depth focus, resulting in suboptimal outcomes.
Insufficient Data Infrastructure: AI thrives on data. Companies might underestimate the infrastructure needed to handle, process, and store vast amounts of data efficiently. Without robust data infrastructure, even the best AI models can falter or provide skewed results.
Employee Pushback: A rapid, all-at-once approach to AI can be unsettling for employees. Fears of redundancy, the challenge of adapting to new technologies, or simply the discomfort of change can lead to resistance. Without proper training, communication, and a sense of inclusion, employees might view AI more as a threat than an asset.
In the race to be AI-first, the tortoise's approach—slow, steady, and thoughtful—often trumps the hare's. AI, while powerful, requires careful integration. The most effective AI-driven transformations are not necessarily the ones that happen the quickest, but the ones that are most strategically aligned, well-planned, and are in sync with the company's capabilities and culture.
The Power of Prioritization
In the journey of AI adoption, knowing what you want to achieve is just half the battle. Equally important, if not more, is understanding which steps to take first. With an array of enticing AI possibilities beckoning, prioritizing which use cases to tackle initially can make the difference between a smooth, successful integration and a muddled, inefficient one.
Introducing the AI Use Case Prioritization Matrix
A structured approach to decision-making can be the guiding light in this process. Enter the AI Use Case Prioritization Matrix—a tool designed to evaluate and rank AI projects based on critical factors:
Feasibility: This evaluates how practical it is to implement a particular AI use case. It considers aspects like the technological challenges involved, the skill sets available in-house, and the financial implications of the project.
Impact: How transformative will the AI project be for the business? Will it lead to substantial cost savings, dramatically enhance user experience, or open up entirely new revenue streams? This dimension gauges the potential value addition of the project.
Data Readiness: AI projects are only as good as the data fueling them. This factor assesses the availability, quality, and relevance of data for the project. Do you have the right data? Is it clean and structured? Are there legal or ethical considerations in using it?
Visualizing these dimensions on a matrix can help stakeholders get a clear picture of where each potential project stands. The ones that rank high on feasibility, impact, and data readiness emerge as the prime candidates for initial implementation.
Practical Examples of Prioritization
Consider a retail business exploring AI for both supply chain optimization and predictive out-of-stock scenarios:
Supply Chain Optimization: - Feasibility: High, as they have a tech-savvy supply chain management team and established relationships with vendors for necessary tools. - Impact: High, since optimizing supply chains can lead to significant cost reductions. - Data Readiness: Moderate, as they have logistical data but it might need cleaning and structuring.
Predictive Analytics for Out-of-Stock Scenarios at Store Level: - Feasibility: Moderate, since while the technology to predict such scenarios exists, integration at every store level might require meticulous coordination and change management. - Impact: High, as predicting out-of-stock scenarios can prevent lost sales, enhance customer satisfaction, and optimize inventory levels. - Data Readiness: High, given that they continuously collect sales and inventory data from each store, which can be used to train predictive models. However, real-time data collection and processing capabilities would be critical.
Given these evaluations, the retail business might still lean towards initiating the supply chain optimization due to its high feasibility and impact. Yet, the potential of the predictive analytics for out-of-stock scenarios, particularly because of its high impact and the availability of data, makes it a compelling subsequent priority. By accurately predicting stock-outs, the retailer can ensure they meet customer demand consistently, fostering loyalty and maximizing sales opportunities.
Prioritization isn't about sidelining certain projects but sequencing them for maximum efficacy. By harnessing the power of prioritization, businesses can embark on their AI journey with clarity, purpose, and a higher likelihood of success.
Building Trust & Familiarity: The 'Small Start'
The journey to AI transformation is as much about technology as it is about people. AI can redefine workflows, change roles, and introduce a novel way of thinking. Given these profound shifts, taking smaller, incremental steps towards AI adoption can often yield better outcomes than a drastic overhaul.
Smaller AI Projects as Introductions for Employees
Starting small serves a dual purpose:
Tangible Demonstrations: Smaller AI projects can act as tangible demonstrations of the technology's potential. Instead of just hearing about AI's capabilities, employees get a hands-on experience, making the concept less abstract and more relatable.
Training Wheels: Just as training wheels help novice cyclists find their balance, small AI projects allow employees to acquaint themselves with new tools and methodologies without feeling overwhelmed. It's an opportunity to learn, make mistakes, and adapt in a relatively low-pressure environment.
The Psychological Aspect: Trust and Fear
AI's entrance into the workplace often comes with a mix of anticipation and apprehension. Two primary psychological challenges arise:
Trust in Technology: Employees might be skeptical about AI's decision-making capabilities, especially if they're used to relying on human intuition. Small projects can gradually showcase AI's accuracy and reliability, fostering trust.
Fear of Redundancy: There's a pervasive fear that AI might replace human jobs. By introducing AI as a tool that augments human capabilities rather than replaces them, and by highlighting roles that AI can't fulfill, businesses can mitigate such fears.
Starting small in AI isn't about thinking small; it's about understanding the human aspect of the AI equation. By focusing on familiarization and trust-building, companies can lay a solid foundation for expansive AI-driven growth in the future.
Resource Optimization & Efficiency
As with any transformative initiative, the incorporation of AI into business processes demands careful resource allocation. Venturing into uncharted territories can be challenging, but by starting small, businesses can optimize their resources, ensuring that every effort counts and laying the groundwork for scaling successfully.
Gaining Insights Through Small Beginnings
Starting with smaller AI projects has the advantage of serving as a litmus test for resource requirements:
Identifying Skill Gaps: By implementing pilot projects, companies can assess if they have the necessary in-house talent or if there's a need for external experts or training programs.
Infrastructure Assessment: Before deploying AI solutions at scale, starting small allows businesses to test their existing IT infrastructure's robustness. This helps in understanding whether it can support AI tools or if upgrades are necessary.
Budgetary Insights: Smaller projects provide a clearer picture of the financial implications, helping businesses prepare better for future investments. It offers a perspective on ROI, making it easier to allocate budgets for larger-scale implementations.
Scaling Strategies: From Pilots to Pervasive AI
Having embarked on the AI journey with pilot projects, the next challenge is scaling these solutions to have a broader impact. Here's how to transition:
Document & Analyze: Post the completion of a pilot, document the process, challenges faced, solutions implemented, and results achieved. This analysis becomes a playbook, guiding larger implementations and preempting potential challenges.
Iterative Expansion: Instead of jumping from a pilot to a full-scale deployment, consider an iterative approach. Expand the pilot's scope step by step, gradually increasing its reach across the organization.
Stakeholder Engagement: Engage with stakeholders at every level. Their feedback can offer valuable insights into making the scaling process smoother. Whether it's the top-level management whose buy-in is crucial for resources or the end-users who interact with the AI solutions daily, every perspective counts.
Infrastructure Evolution: As you scale, ensure that the IT infrastructure evolves in tandem. This might mean more robust data storage solutions, faster processing capabilities, or enhanced security measures.
Starting small in AI does more than just simplify the process. It brings clarity, provides direction, and ensures that resources – whether human, technological, or financial – are optimized to drive maximum efficiency and impact.
Feedback Mechanism and Iterative Learning
In the dynamic world of AI, evolution is continuous. One of the most potent tools for this evolution is feedback. The adoption of AI, especially when initiated on a smaller scale, provides fertile ground for learning, refining, and continuously enhancing AI projects. By constructing a robust feedback mechanism and embracing iterative learning, businesses can ensure their AI solutions not only remain relevant but also continue to deliver optimal results.
Using Early Projects as a Testing Ground
Smaller, preliminary AI projects serve multiple purposes:
Proof of Concept: These projects validate the feasibility and potential impact of AI in specific operational areas.
Safe Environment for Risks: Given their limited scale, early projects offer a safer environment to experiment, test hypotheses, and take calculated risks without large-scale repercussions.
Immediate Feedback Loop: Because of their focused nature, initial projects can provide quick feedback, allowing teams to adjust strategies in real-time.
Incorporating Feedback for Future Refinements
A well-constructed feedback loop can be the cornerstone of AI project enhancement:
User Experience Feedback: Gather insights from end-users interacting with the AI solution. Their on-ground experiences can highlight usability issues, areas for improvement, or potential new features.
Technical Feedback: Constantly monitor the AI system for glitches, inaccuracies, or inefficiencies. Technical feedback ensures that the system stays updated, secure, and efficient.
Performance Metrics Evaluation: Establish clear metrics to evaluate the AI system's performance. Regularly comparing actual outcomes with projected results can provide insights into the system's efficacy and areas needing attention.
Iterative Development Process: Instead of viewing AI projects as one-off implementations, adopt an iterative development mindset. With each feedback cycle, refine the solution, re-deploy, and gather more feedback. This continuous cycle ensures the AI system remains adaptable and up-to-date.
Cultivate a Feedback-friendly Culture: Encourage a company culture where feedback, both positive and constructive, is valued. By ensuring stakeholders at all levels feel comfortable sharing their observations, businesses can harness a wealth of knowledge to enhance their AI endeavors.
In conclusion, the feedback mechanism is not just an evaluative tool but a powerful driver for growth and innovation. By coupling iterative learning with consistent feedback, companies can pave the way for AI solutions that are resilient, relevant, and robustly aligned with their evolving needs.
Conclusion
As we navigate the vast and exciting landscape of AI, it's evident that while ambition propels us forward, strategy anchors us. The allure of AI's transformative power can sometimes lead businesses to a sprint, but the journey to AI excellence is more a marathon. A phased, deliberate, and strategic approach not only mitigates risks but also ensures that each step is built on a solid foundation of learning and insights.
Dreaming big about the future is crucial, for it charts our direction. Yet, as with any journey, it's the individual steps that count. In the world of AI, each of these steps should be taken with careful consideration, ensuring that while the gaze remains on the horizon, the immediate path is navigated with caution and foresight. Remember, in the race to AI mastery, it's not just about speed but also about stamina, resilience, and adaptability.
Sumo Analytics is a data science and AI laboratory, specializing in the realm of prediction science. We build and deploy advanced AI systems that elegantly marry human intelligence with the computational power of artificial intelligence, enabling our clients to achieve unparalleled performance.