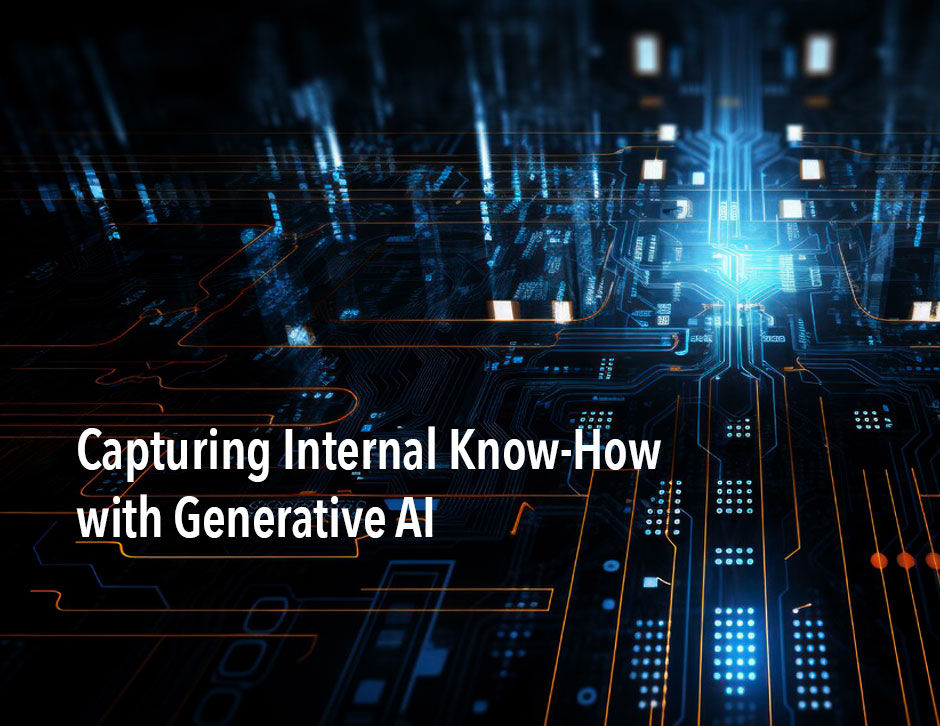
Across the diverse landscapes of modern organizations, a significant challenge continually emerges: a considerable portion of internal knowledge is inaccessible, hidden within documents, reports, and the expertise of employees. Traditional knowledge management systems, with their reliance on manual search and categorization, often fall short in unlocking this treasure trove of insight and experience. As organizations grow and evolve, the need to effectively capture, preserve, and make accessible this wealth of internal know-how becomes not just a priority but a necessity for sustained innovation and competitive advantage. Generative AI is a transformative technology that is redefining the paradigms of knowledge management. By combining advanced search functionalities with intuitive chat interfaces, Generative AI offers a novel approach to interacting with and leveraging the depths of organizational knowledge.
The Critical Need for Capturing Internal Know-How
The ability to effectively manage and leverage internal knowledge stands as a cornerstone of organizational resilience and innovation. Despite this, many organizations find themselves at a disadvantage, struggling to capture the full spectrum of internal know-how that is essential for staying ahead in competitive markets. This failure not only represents a missed opportunity for growth but also poses a risk to maintaining relevance and operational efficiency.
The crux of the challenge lies in the transient nature of employee knowledge and the siloed storage of critical information. With workforce mobility on the rise, the knowledge possessed by an individual often departs with them, leaving a gap that is difficult to fill. Moreover, even when attempts are made to document and store this valuable know-how, it frequently ends up in disparate databases or document management systems, where it becomes obscured and, over time, forgotten.
Generative AI is poised to change this narrative by facilitating a more dynamic and effective approach to knowledge management. It enables organizations to capture, store, and make accessible the vast amounts of unstructured data—emails, documents, presentations—that traditionally remain underexplored. By applying advanced AI techniques, companies can now convert these reservoirs of passive data into active, searchable, and interactive knowledge bases. This transformation is not just about preserving information; it’s about making it a living part of the organizational ecosystem, easily accessible and continuously enriched through employee interaction.
Moreover, the integration of Generative AI into knowledge management practices offers a unique opportunity to bridge the gap between knowledge capture and knowledge utilization. Employees can engage in natural language dialogues with the system, asking complex questions and receiving answers that synthesize information from across the entire organization. This not only enhances decision-making and problem-solving but also fosters a culture of learning and knowledge sharing among employees.
The critical need for capturing internal know-how, therefore, cannot be overstated. As organizations navigate the complexities of the digital age, the ability to effectively manage internal knowledge becomes a key differentiator. Generative AI represents a pivotal tool in this endeavor, transforming static repositories of information into dynamic sources of insight and innovation, and ensuring that the collective knowledge of an organization is more than just stored—it is actively leveraged for continuous growth and success.
Combining Chat and Search with Generative AI
The introduction of Generative AI (GenAI) technologies marks a significant shift in knowledge management, integrating chat and search functionalities in a way that enhances how we interact with and access organizational knowledge. This integration moves us away from the limitations of traditional search tools, offering a more dynamic and user-friendly experience.
The Evolution of Search
Historically, accessing internal knowledge has been predominantly reliant on keyword-based search tools that necessitate precise input to yield relevant results. This method, while functional, often leads to challenges in discovering information if the exact terminology or the existence of specific documents is unknown. The linear and sometimes rigid nature of traditional search tools can inhibit the discovery of related insights and the exploration of broader content themes.
The Emergence of Conversational Interfaces
In contrast, conversational interfaces, powered by GenAI, introduce a more intuitive way of interacting with data. Users can pose questions or describe their information needs in natural language, akin to a dialogue with a colleague. This method leverages the AI's understanding of context, intent, and the nuances of human language, offering responses that are not only relevant but also cognizant of the user's underlying objectives.
The Power of Combination
By combining chat and search capabilities, GenAI creates a synergistic tool that enhances knowledge discovery and access. This combination allows users to:
Engage in Natural Dialogues: Users can have conversational interactions with the AI, asking follow-up questions, clarifying previous inquiries, or exploring tangential topics, much like they would in a human conversation.
Experience Contextual and Semantic Search: The AI understands the context of queries and can perform semantic searches, looking beyond the literal match to find content that matches the query's intent and meaning.
Discover Hidden Insights: The integrated approach enables the AI to draw connections between disparate pieces of information, uncovering insights that would be difficult to find through traditional search methods.
Access Tailored Summaries: Instead of merely pointing to documents, the AI can summarize content, highlight relevant sections, and even generate new insights based on the amalgamation of various data sources.
Implementation in Practice
For organizations, the practical implementation of this combined approach means transforming their knowledge bases into dynamic resources that are more accessible and useful to employees. It involves indexing vast amounts of unstructured and structured data, training the AI on organizational content, and continuously refining the system based on user interactions and feedback.
The result is an AI-driven knowledge management system that not only stores information but actively facilitates its discovery and use, making every search or conversation a step towards deeper understanding and more informed decision-making.
In essence, the integration of chat and search functionalities via Generative AI represents a significant leap forward in how organizations manage and interact with their internal knowledge. It transforms the quest for information from a mere retrieval process into an organisational process and culture of discovery, making knowledge not just available but truly accessible.
Knowledge Mining: Unearthing Insights from Local Documents and Data
While the primary focus of leveraging Generative AI in knowledge management has been on enabling intuitive, conversational access to an organization's wealth of documents, there exists a complementary layer that significantly enhances this interaction: knowledge mining. This advanced facet of AI technology goes beyond the immediate retrieval of information, diving deeper into the vast array of local documents and data to uncover hidden patterns, relationships, and insights that might not be immediately apparent.
Knowledge mining acts as the intelligent underpinning of the conversational interface, analyzing unstructured and semi-structured data to enrich the knowledge base from which the AI draws its responses. This dual approach ensures that when employees engage in a dialogue with their internal knowledge system, they are not only accessing direct information but are also presented with synthesized insights drawn from a comprehensive analysis of the organization’s entire data spectrum.
By integrating knowledge mining, we enhance the GenAI’s ability to provide contextually rich, insightful answers that are informed by a deeper understanding of the organization's knowledge landscape. This synergy between conversational access and analytical depth creates a more powerful and effective knowledge management tool, capable of supporting both immediate information needs and strategic decision-making.
Understanding Knowledge Mining
At its core, knowledge mining employs a suite of intelligent services to analyze data deeply. It utilizes natural language processing (NLP) to interpret and extract information from text-based documents, optical character recognition (OCR) to convert images and scanned documents into searchable text, and machine learning algorithms to identify trends and patterns across all types of data.
The Application in Organizational Data
Organizations typically possess a wealth of data in various formats, including reports, emails, presentations, and more. Knowledge mining enables the transformation of this data into actionable insights. For instance, it can reveal operational inefficiencies, uncover market trends, and provide a deeper understanding of customer behaviors. Moreover, it can make previously inaccessible information readily available to employees, empowering them with the knowledge to make informed decisions.
Enhancing Decision-Making and Innovation
By unlocking the value hidden within data, knowledge mining contributes significantly to more informed decision-making and fosters a culture of innovation. Organizations can identify new opportunities, anticipate market changes, and tailor strategies to meet evolving customer needs. Furthermore, knowledge mining supports a more agile response to internal and external challenges by providing a richer, data-driven context for strategic initiatives.
Implementation Challenges and Opportunities
Implementing knowledge mining requires a thoughtful approach to data governance, privacy, and security. Organizations must ensure that they adhere to data protection regulations and ethical guidelines while harnessing the power of their data. Despite these challenges, the opportunities presented by knowledge mining for enhancing organizational knowledge, improving efficiency, and driving growth are immense.
Knowledge mining is not just about analyzing data; it's about transforming data into a strategic asset. By leveraging AI and machine learning to unearth insights from data, organizations can navigate the complexities of the modern business environment more effectively, making knowledge mining an indispensable tool in the era of information.
Retrieval-Augmented Generation (RAG) Explained
In the journey towards more intuitive and insightful knowledge management systems, Retrieval-Augmented Generation (RAG) stands out as a pivotal technology. RAG uniquely combines the best of two worlds: the deep, contextual understanding of language models with the precision of information retrieval systems. This hybrid approach enables organizations to not only find and access documents more efficiently but also to understand and utilize the knowledge within them in transformative ways.
The Mechanics of RAG
RAG operates by first identifying the most relevant pieces of information from a vast dataset in response to a query. This retrieval step is crucial and is powered by algorithms designed to search through documents, pinpointing the data most likely to be relevant to the user's request. Once this data is retrieved, the second phase begins, where a generative model uses the retrieved information to construct a coherent, contextually appropriate response.
This process mirrors a natural human approach to answering questions: finding relevant information and then synthesizing it into a comprehensive answer. By automating this process, RAG models provide a powerful tool for accessing and leveraging organizational knowledge.

Enhancing Conversational Interfaces
The RAG technology significantly boosts the capabilities of conversational interfaces in knowledge management systems. Users can ask complex, nuanced questions and receive answers that are not just retrieved but also generated based on a wide array of relevant information. This capability makes the interaction with the system more natural and effective, closely resembling a conversation with a knowledgeable human colleague.
The Impact on Knowledge Discovery
RAG's ability to blend retrieval with generation has profound implications for knowledge discovery within organizations. It enables employees to uncover insights that would be challenging to find through traditional search methods or direct querying alone. By facilitating access to a broader range of information and generating responses that weave together various data points, RAG helps organizations make better use of their internal knowledge, fostering innovation and informed decision-making.
Implementation Considerations
Deploying RAG within an organization's knowledge management system involves careful consideration of data sources, indexing strategies, and the training of the AI model to ensure relevance and accuracy. Privacy and security measures must be paramount, given the sensitive nature of the information being processed. Furthermore, user feedback is invaluable for refining the model, ensuring that it continually improves in delivering precise and useful responses.
Retrieval-Augmented Generation represents a significant step in the evolution of knowledge management technologies. By enabling more sophisticated, nuanced interactions between employees and the organization's knowledge base, RAG offers a path towards not just more efficient information retrieval, but a deeper, more insightful exploration of the wealth of knowledge that organizations possess.
Implementing AI-Driven Knowledge Management
The integration of AI technologies, including Generative AI and knowledge mining, into knowledge management systems presents a promising avenue for organizations to enhance their information access and insight generation capabilities. However, successful implementation requires strategic planning, technological readiness, and an understanding of the organizational impact. Here's a guide to navigating the complexities of adopting AI-driven knowledge management solutions.
Assessing Organizational Needs and Readiness
The first step in implementing an AI-driven knowledge management system is a thorough assessment of organizational needs and readiness. This involves identifying the specific challenges and opportunities within the current knowledge management practices and evaluating the technological infrastructure and data governance policies in place. Understanding the gaps and potential areas of improvement will help tailor the AI solution to address specific organizational requirements effectively.
Choosing the Right Technologies
Selecting the appropriate AI technologies is crucial for meeting the organization's unique needs. This decision should be based on the types of data the organization deals with, the desired functionalities of the system (e.g., conversational interfaces, deep search capabilities, insight generation), and the integration capabilities with existing IT infrastructure. A combination of Generative AI for natural language processing and interaction, along with RAG for enhanced retrieval and generation, can offer a comprehensive solution.
Data Preparation and Governance
A key aspect of implementing AI-driven knowledge management is preparing the data that will feed into the AI models. This involves organizing, cleaning, and annotating data to ensure its quality and relevance. Additionally, establishing robust data governance practices is essential to address privacy concerns, secure sensitive information, and comply with relevant regulations.
Training and Customization
To maximize the effectiveness of AI in knowledge management, the selected models need to be trained on organizational data. This training process involves customizing the models to understand the specific language, terminology, and context of the organization. Continuous learning mechanisms should also be in place to allow the system to evolve and improve over time based on user interactions and feedback.
Change Management and User Adoption
Implementing a new knowledge management system can significantly change how employees access and interact with organizational knowledge. A comprehensive change management strategy is necessary to facilitate smooth adoption, including training programs to familiarize employees with the new system, ongoing support to address questions and issues, and mechanisms to collect user feedback for continuous improvement.
Measuring Success and Iterative Improvement
Finally, establishing metrics to evaluate the success of the AI-driven knowledge management system is critical. These metrics might include user engagement rates, the accuracy of information retrieval, user satisfaction scores, and the impact on decision-making and innovation. Regular reviews of these metrics can inform iterative improvements to the system, ensuring it continues to meet the evolving needs of the organization.
The Future of Knowledge Management with AI
AI-driven knowledge management is not just an incremental improvement over existing systems; it's a paradigm shift that redefines how organizational knowledge is captured, accessed, and utilized. Here's what the future may hold.
Predictive and Proactive Knowledge Delivery
The future of knowledge management will likely see AI systems not just responding to queries but anticipating the information needs of users based on their roles, tasks, and historical interactions. AI could proactively provide insights, suggest resources, and even alert employees to emerging trends and patterns before they become explicit knowledge needs. This predictive capability will transform knowledge management from a reactive to a proactive tool in the hands of employees.
Enhanced Collaborative Intelligence
AI will further blur the lines between human and machine intelligence, leading to a more collaborative relationship where AI systems offer not just information retrieval but also contribute to knowledge creation. Through the analysis of vast amounts of data and the identification of connections that might not be immediately obvious to human researchers, AI can become a co-creator of knowledge, enhancing the collaborative intelligence within organizations.
Seamless Integration Across Platforms
The integration of AI-driven knowledge management systems across various work platforms and tools will become more seamless, ensuring that knowledge access is not siloed but ubiquitously available, regardless of the digital environment employees are working in. This integration will ensure that knowledge flows freely across organizational boundaries, making it accessible whenever and wherever it's needed.
Ethical and Secure Knowledge Utilization
As AI becomes more embedded in knowledge management, ethical considerations and data security will take center stage. Future systems will need to balance the power of AI with the responsibility of handling sensitive information, ensuring that knowledge is used ethically and that user privacy is respected. This will involve not just technological solutions but also organizational policies and practices designed to safeguard data and ensure its ethical use.
Lifelong Learning and Organizational Agility
Finally, AI-driven knowledge management will support the concept of lifelong learning within organizations. By making knowledge more accessible and engaging, employees will be encouraged to continuously learn and adapt, fostering a culture of curiosity and innovation. This will not only benefit individual employees but also enhance the overall agility and resilience of organizations, enabling them to navigate the complexities of an ever-changing business landscape more effectively.
xxx
The integration of Generative AI into knowledge management marks a significant step towards transforming the accessibility and utility of organizational knowledge. By blending advanced search capabilities with conversational interaction and enriching this experience with insights from knowledge mining and RAG technologies, these AI-driven systems not only make information retrieval more intuitive but also unlock the deep value hidden within vast data repositories. This evolution represents not merely an enhancement of existing processes but a foundational shift towards a more informed, agile, and innovative organizational culture.
Sumo Analytics AI is a pioneering AI laboratory that combines advanced AI technologies with human insight to optimize operations and drive superior performance. Our approach focuses on creating intelligent decision-making systems, utilizing the latest in AI research to produce tangible impacts. We specialize in developing and deploying human-centric AI solutions, enabling our clients to achieve unmatched operational excellence.